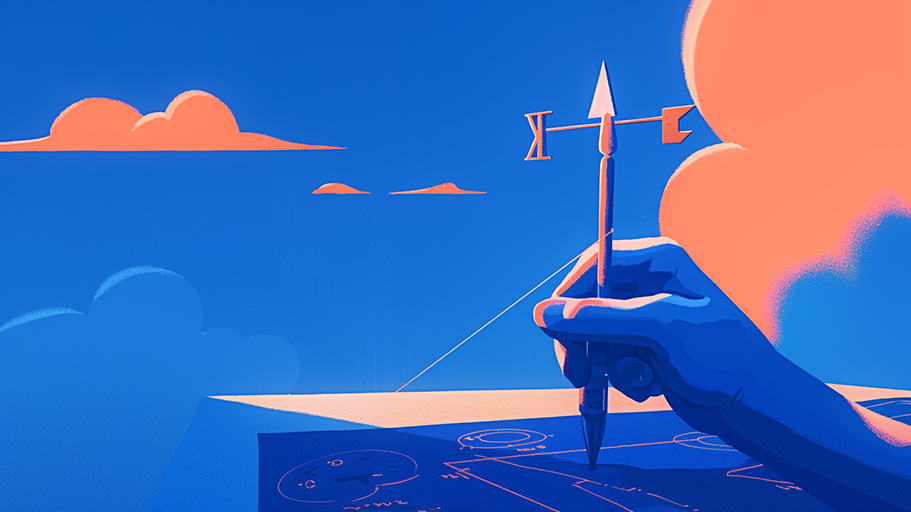
The year 2022 saw significant advancements in the realm of Artificial Intelligence(AI). Natural Language Processing, Image Generation, Machine Learning, and other related fields have all made remarkable strides, making AI tools widely used across various domains. In the first half of 2022, a large number of generative AI models emerged, which greatly attracted the attention of the research community and the public. Soon after, ChatGPT was born, directly pushing people’s enthusiasm for AI to its climax. While the whole world marvels at the swift progress of AI, researchers are tirelessly pushing the boundaries of AI algorithms behind the scenes, making continuous efforts in general foundation models, AI for Science, multi-modality, AI applications, etc. Groundbreaking work is constantly emerging. We have summarized the top 10 most influential works on AI in 2022 as a witness to this extraordinary year for AGI.
1. Flamingo
The Flamingo is a multimodal multitask model with in-context learning capabilities proposed by DeepMind. Like GPT-3, it can solve a wide variety of complex multimodal tasks by providing a small number of specific examples of the model (multimodal few-shot). To achieve multimodal in-context learning, Flamingo has made several innovations:
- The Flamingo “embeds” visual information into a pre-trained large language model, enabling the robust sequential reasoning ability of the language model to perform reasoning tasks on multimodal data, which not only saves huge training costs but also makes full use of the merit of the current language model;
- The Flamingo can handle sequences of arbitrarily interleaved visual and textual data, giving it powerful multimodal in-context learning capabilities;
- The Flamingo model uses the Perceiver-based architecture, which can unify the information of video and image into one input space, solving the problem of the difference in the length distribution of image and video in various scenes.
Apart from all that mentioned above, the Flamingo shows incredible performance. Under the few-shot methods, it outperforms other SotA models on 16 multimodal tasks and even beats the SotA fine-tuned model on 6 tasks. The advent of the Flamingo represents that the field of multimodal research has begun to embrace the in-context learning paradigm, which greatly propelled the development of multimodal research.
Related article: https://arxiv.org/abs/2204.14198
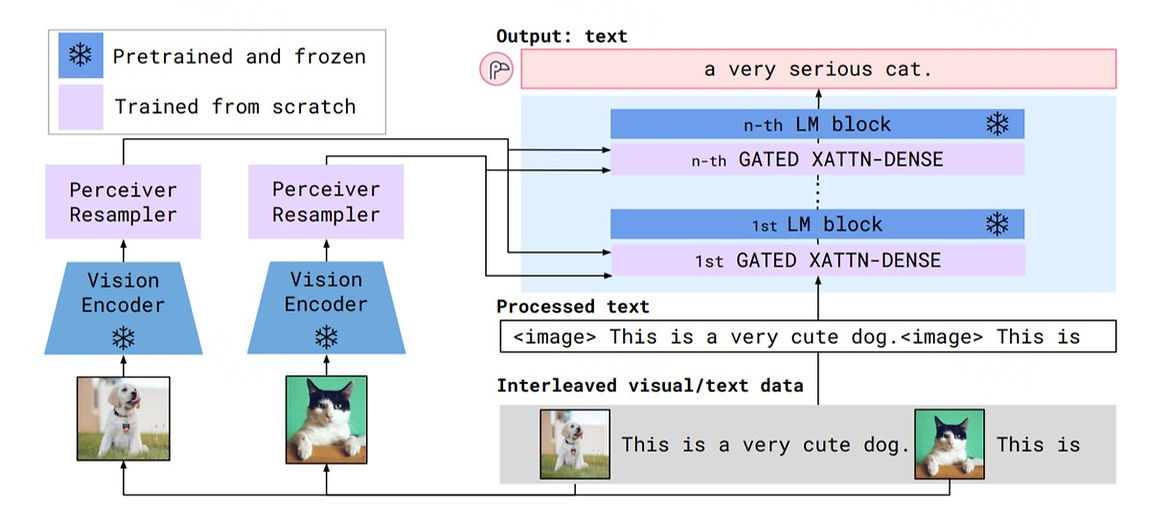
2. Gato
Gato is a “generalist” AI agent designed by DeepMind. It’s this idea that all data, including language, images, game operations, action buttons, joint torques, etc., can be serialized into a flat sequence. Unified modeling can therefore be done through autoregressive models. Inspired by this, Gato uses autoregressive models for sequence learning on 604 different tasks with different modalities and information representations, thus avoiding the need to make a policy model with appropriate inductive biases for each different task. Meanwhile, it increases the diversity of data and training samples, so that the model can learn more general representations and task patterns, which improves the versatility of the model.
Related article: https://arxiv.org/abs/2205.06175
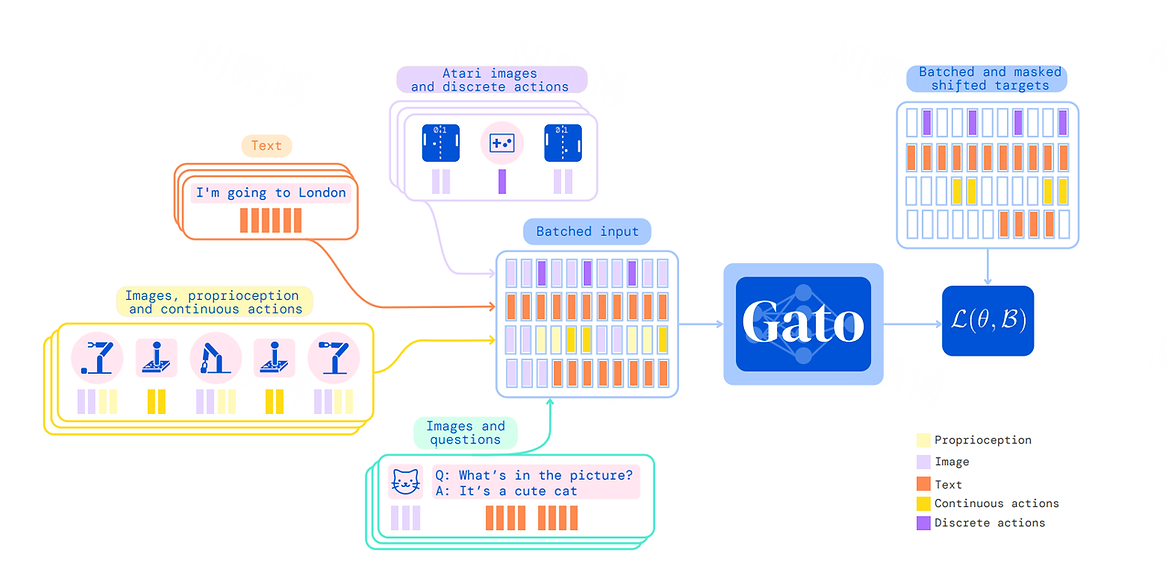
3. ChatGPT
As soon as ChatGPT came out, it refreshed everyone’s understanding of AI chatbots and attracted animated discussion worldwide. ChatGPT and InstructGPT employ similar underlying methodologies, although there are some distinct aspects related to the training data and the specific versions of GPT employed. In particular, ChatGPT leverages the GPT-3.5 series during its training phase and deploys slightly divergent datasets.
What deserves to be mentioned is that ChatGPT uses a technology called Reinforcement Learning from Human Feedback (RLHF), which trains the Rank model as a reward model through manual data labeling. Over time, the model can learn which responses are “better” so that its responses can gradually align with human-labeled ones.
ChatGPT’s appearance has led to a paradigm shift in the application of large language models. Due to its powerful instruction-following ability, ChatGPT generally does not require complex “prompt engineering” to induce reasonable responses. Instead, users can easily input instructions in natural language.
Related article: https://arxiv.org/abs/2203.02155
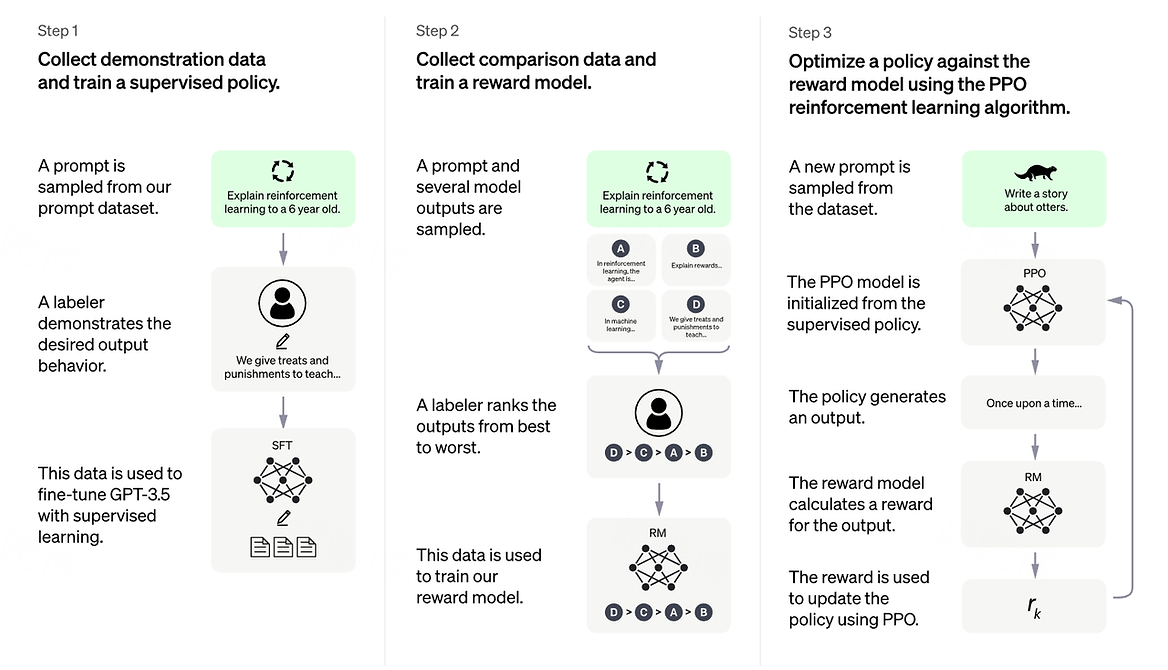
4. LeCun’s Autonomous AI Architecture
According to LeCun, an autonomous agent should be a set of “Macro Architecture” composed of various functional modules, rather than a simple foundation model. With that in mind, LeCun put forward an autonomous AI architecture that incorporates multiple sub-modules, drawing inspiration from the biological brain.
LeCun provided some principled theories and hypotheses to guide the implementation of his architecture. Although he did not give the answers to every detail of the modules, he contributed his own conjectures and possible implementation methods for the learning mechanism, utilization of the world model, the overall design of the architecture, and the intrinsic motivation system that gives the system autonomy. This work serves as a valuable reference within the broader landscape of general artificial intelligence research.
Related article: https://openreview.net/pdf?id=BZ5a1r-kVsf
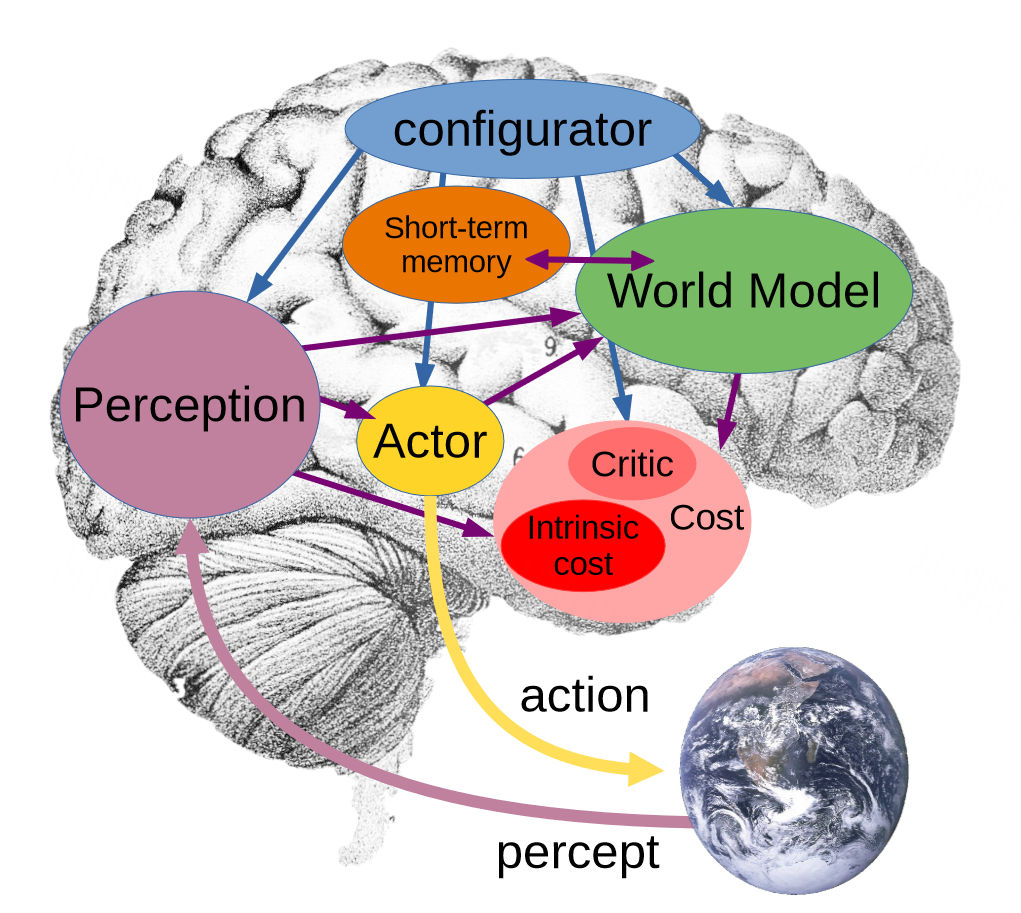
5. OPT
While the progress made in large language models is exhilarating, the inability to directly access these models greatly limits researchers from exploring these models’ limitations and potential risks. The OPT is Meta’s replication of GPT-3, possessing the same 175 billion parameters. The most distinctive characteristic of OPT lies in its openness. Meta has released the entire OPT model as open source, including its weights, training code, deployment code, and training process, thereby offering unprecedented transparency and openness for the development and research of language models.

6. Stable Diffusion
Artificial Intelligence Generated Content(AIGC) is one of the most trending topics in 2022, owing its origins to the development of diffusion models. These models serve as the backbone of contemporary Text2Image modeling techniques. Two of the most renowned and extensively employed Text2Image models – DALL-E2 and Stable Diffusion – have harnessed the power of diffusion models to remarkable effect.
Stable Diffusion based on Latent Diffusion Models, enabling the swift production of images that align seamlessly with a given textual description. Different from DALL-E2, Stable Diffusion is completely open source. It provides a model that can be quickly adjusted and applied so that it can be integrated into a vast array of downstream applications as a functional component, thereby substantially broadening the scope of Text2Image’s applications.
Related article: https://arxiv.org/abs/2112.10752
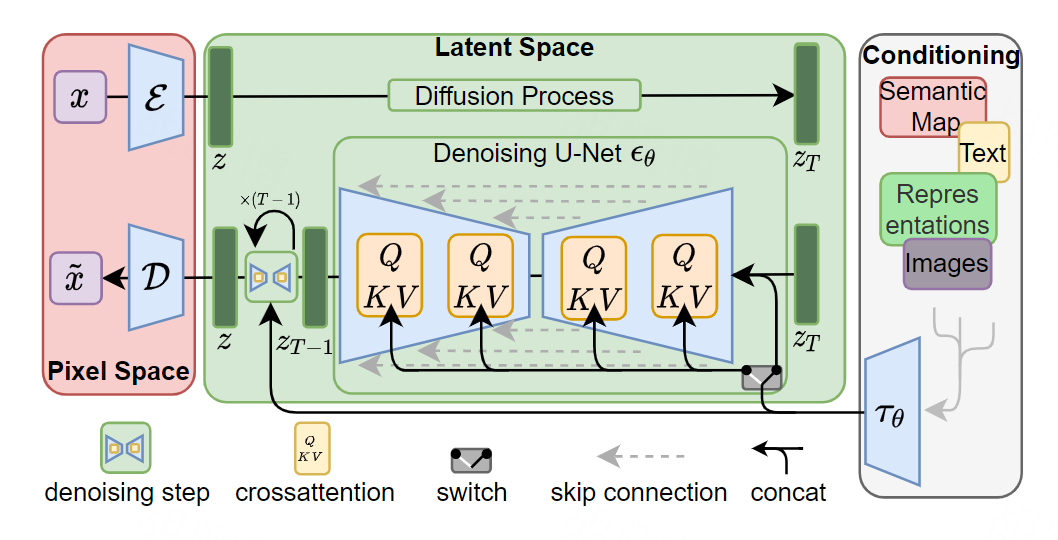
7. Alphacode
Large language models have made great progress in text generation and comprehension, yet their ability in problem-solving is still confined to relatively simple mathematical and programming problems. DeepMind designed AlphaCode, a model that can write complex computer algorithm programs to solve novel problems that require a blend of skills involving critical thinking, logic, algorithms, coding, and natural language comprehension. Additionally, AlphaCode has been reported to perform better than 54% of the players in programming competitions, marking the first time an AI system has achieved a competitive level of performance in such competition. As Alphacode continues to evolve, it can effectively help programmers improve productivity in the future.
Related article: https://www.science.org/doi/10.1126/science.abq1158
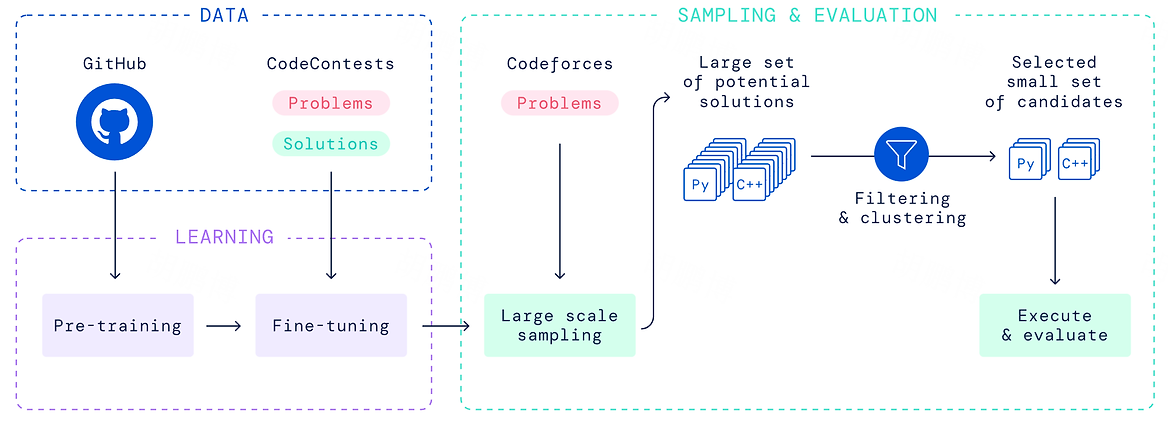
8. Chain of Thought
With the rise of Prompt Learning, the research community began to pay attention to Prompt Engineering, and the Chain of Thought(CoT) stands out as one of the most interesting findings in this boom. The CoT was proposed by Jason Wei from Google Brain. It uses discrete prompts, and its core method is to write the reasoning process concurrently with the sample prompt. This step “prompts” the model that when outputting the answers, it also needs to output the process of deriving the answer, which greatly improves the accuracy in performing reasoning tasks. Researchers have significantly expanded this method, and CoT has been extensively employed in various language-model-based reasoning tasks, leading to significant improvements in language models at complex reasoning tasks.
Related article: https://arxiv.org/abs/2201.11903
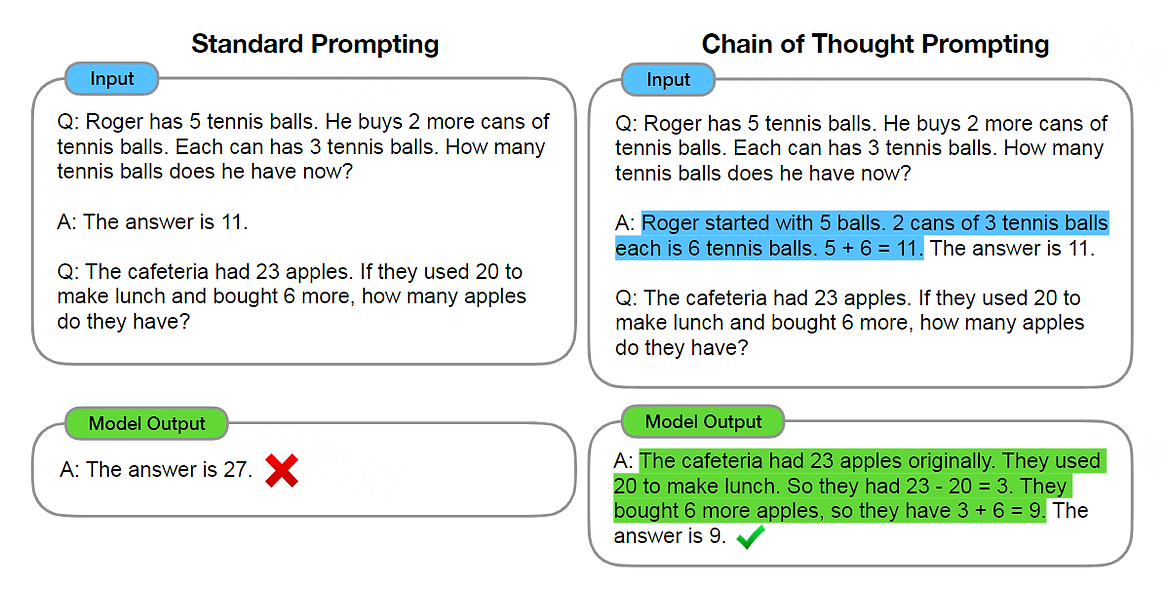
9. CICERO
Diplomacy is a multiplayer competitive game of diplomacy and is set in Europe in 1901. Each of the 7 players in the game assumes control of a country, and through cooperation and negotiation, they try to occupy as much territory as possible. CICERO is a system composed of a dialogue engine and a strategic reasoning engine. It pretended to be a real player and joined the game. A total of 82 human players were unable to detect that they were playing against an AI system in 40 anonymous online games. And just as a fact, its average score reached 25.8%, more than twice the average score of human players, ranking in the top 10% of players who participated in more than one game. Although previous research has made great progress in training AI systems to imitate human language, building AI systems that can use language to negotiate, persuade, and cooperate with humans in achieving strategic goals remains a major challenge. CICERO has taken a big step forward in this regard.
Related article: https://www.science.org/doi/10.1126/science.ade9097
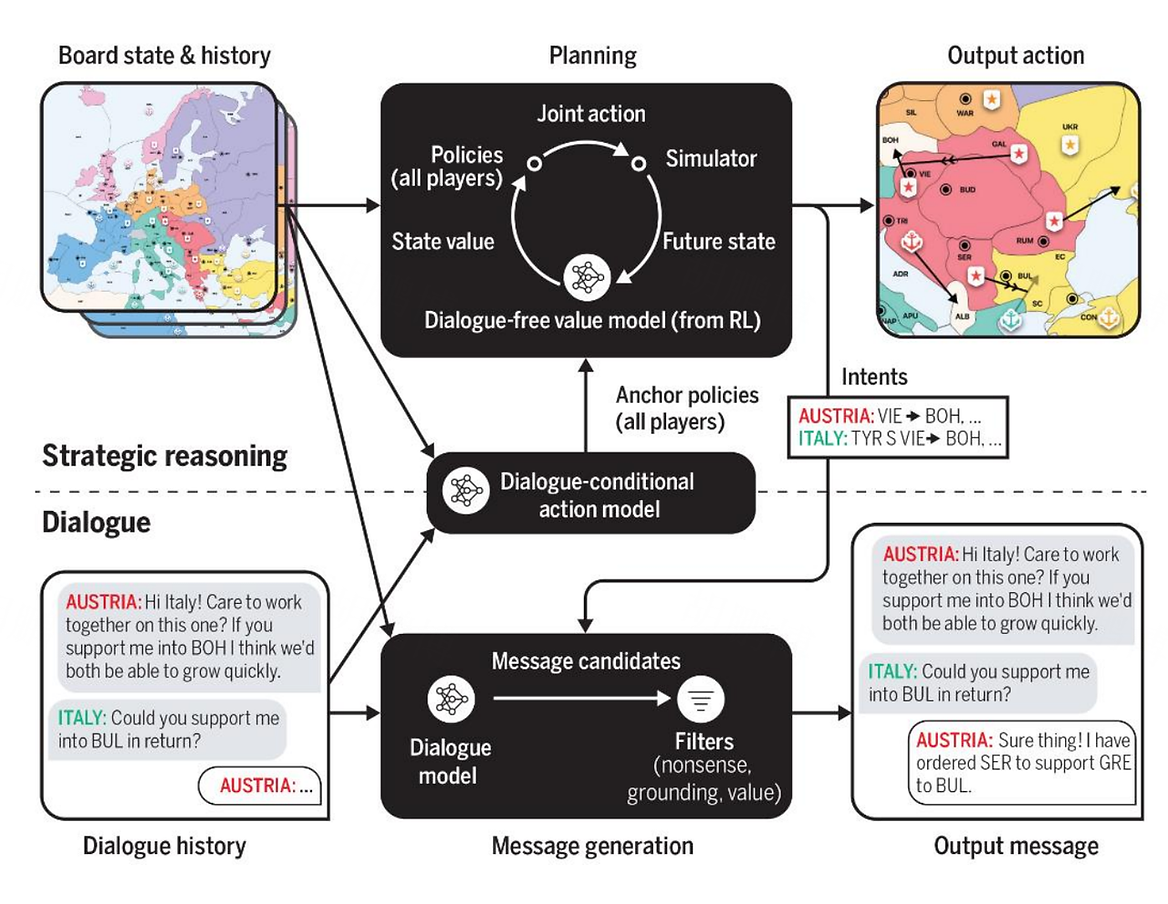
10. Minerva
Minerva’s deep learning language model can solve mathematical and scientific problems through step-by-step reasoning. Its solutions include numerical calculations and symbolic manipulations without relying on external tools such as calculators. By collecting a large amount of training data related to quantitative reasoning problems and using advanced reasoning techniques, the model has achieved significant improvements on various difficult quantitative reasoning tasks. Although the performance of the model is still far lower than that of humans, it narrows the gap in the application of language models for solving university-level mathematics, science, and engineering problems. If the model can be further improved, it has the potential to exert substantial social influence.
Related article: https://arxiv.org/abs/2206.14858
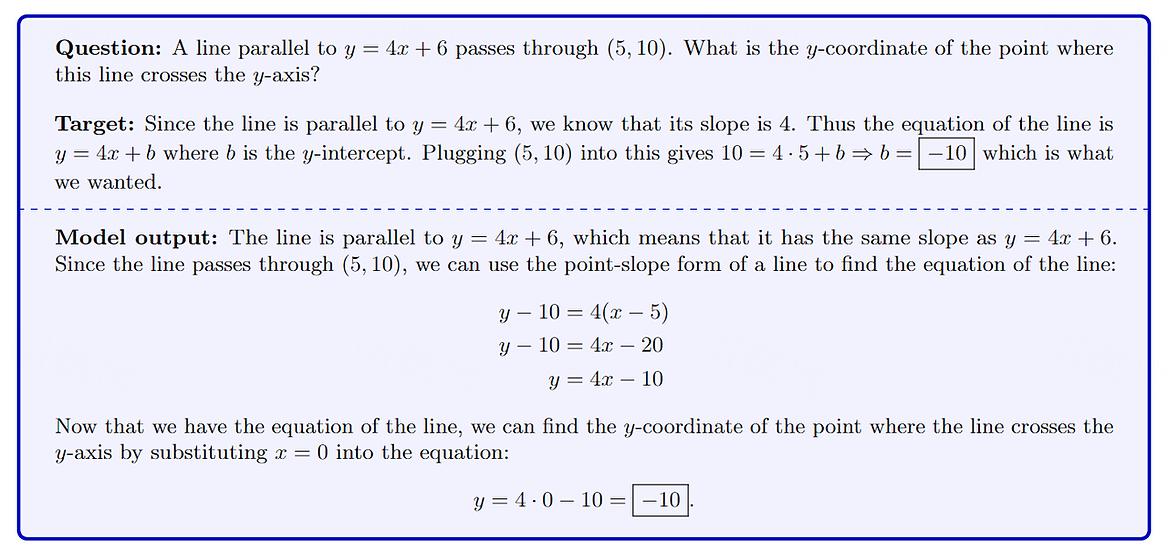
Related Reading:
AI Agents Explained: A Beginner’s Guide
How to Boost Productivity with AI Agents
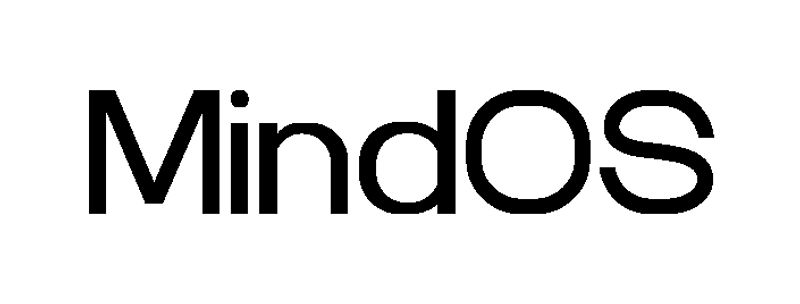
Leave a Reply