AI Agents vs AI Chatbots: Who Will Lead the Future?
Updated: Feb 20

In today's AI-driven world, chatbots have emerged as indispensable companions in our daily lives. AI Chatbots are designed to facilitate conversations and provide information. However, conversion is often just a means to an end. Most of the time, the purpose of conversation is to get things done, which goes beyond the capabilities of traditional chatbots. This is where AI agents come into play. While chatbots excel at fulfilling our communication and expression needs, AI agents take it a step further by focusing on accomplishing tasks and achieving goals. These sophisticated systems harness the power of artificial intelligence algorithms to perform complex actions and automate various processes. AI agents can handle a wide range of tasks, such as making reservations, managing schedules, conducting financial transactions, and much more. Although some may consider LLM-based chatbots as a subset of AI agents, it is crucial to differentiate between the two. AI agents have a broader scope, encompassing various forms of intelligent systems that go beyond conversational interfaces. In this article, we'll see what characteristics AI agents possess that would set them apart from chatbots, their application scenarios, and how they can synergize organically.
Exploring AI Chatbots
The earliest chatbot, known as ELIZA, was developed in 1966 at the MIT Artificial Intelligence Lab. ELIZA was designed to simulate the renowned psychiatrist Carl Rogers. It operates on a predefined set of rules and patterns. Since then, chatbots have adopted this traditional rule-based form. Rule-based chatbots follow a specific flowchart or decision tree to respond to user inputs. They rely on a fixed set of rules and patterns that are programmed by developers in advance. Such chatbots can offer 24/7 service to users and can provide accurate responses within the scope of their predefined rules. However, when faced with complex or ambiguous queries, they seem to be at a loss.
To solve these problems, LLM-based chatbots, also known as generative chatbots, have been developed. These chatbots leverage advanced machine learning techniques, such as deep learning and natural language processing (NLP). They are trained on large datasets to understand and generate human-like responses. They use language models, such as GPT (Generative Pre-trained Transformer), to generate contextually relevant and coherent responses. LLM-based chatbots have the advantage of understanding context and generating more natural and dynamic responses. Due to extensive data and ample computational resources for training and inference, AI chatbots can handle a wider range of queries and adapt to different conversational styles.
Meeting AI Agents
AI Agents, on the other hand, are autonomous entities that can perform tasks or make decisions without constant human intervention. They are often used in complex systems or environments where real-time decision-making is required. AI Agents can analyze large volumes of data, adapt to changing conditions, and take actions autonomously. With specific designs, agents can continue to learn and update themselves in the environment over time. Additionally, they can collaborate with other agents or systems, enabling them to tackle complex tasks that require coordinated efforts.
In the previous blog, we provided a beginner-friendly introduction to AI agents, offering a comprehensive yet easy-to-understand overview. Recently, we aim to delve deeper into this topic by presenting a more detailed report. Thus, in this article, we will focus more on the differences between AI agents and AI chatbots.
AI Agents vs AI Chatbots: Functionality Comparison
Conversation-oriented Chatbots vs Goal-oriented Agents
As their names suggest, AI chatbots are conversation-oriented and AI agents are goal-oriented. While AI chatbots are primarily focused on engaging in conversations with users and providing information, AI agents are designed to perform specific tasks.
AI chatbots are designed to simulate human-like conversations and interact with users through natural language processing. They excel at understanding and responding to user queries, leveraging techniques such as sentiment analysis, entity recognition, and text classification. Their main goal is to provide accurate and contextually relevant information to users in a conversational manner.
AI agents are designed to perform specific tasks or functions autonomously, with the ultimate aim of achieving a particular objective. This goal-oriented nature allows AI agents to operate in complex and dynamic environments, making decisions and taking actions to accomplish their designated tasks. When faced with a task, AI agents actively split, plan, and execute the necessary steps to accomplish the goal. Unlike passive information providers, AI agents involve taking tangible steps to achieve desired outcomes. An essential characteristic of AI agents is their underlying motivations, which guide their behavior. These motivations can be based on various factors, such as optimizing efficiency, achieving specific objectives, or maximizing user satisfaction. By self-motivating, AI agents are able to prioritize tasks, allocate resources, and make decisions that align with their designated goals.
Memory
These two AI forms also differ in memory abilities. AI chatbots typically rely more on short-term memory. They store information temporarily during a conversation to maintain context and provide relevant responses. Once the conversation ends, the short-term memory is often cleared to make room for new interactions. This approach helps chatbots focus on immediate conversation without retaining unnecessary information.
AI agents could have long-term memory capabilities, enabling them to retain information over extended periods. This long-term memory allows them to accumulate knowledge and learn from past experiences, which can be valuable for improving their performance and decision-making abilities.
Moreover, AI agents can have user-specific memory to personalize interactions. They can remember user preferences, past queries, or specific details shared during the conversation. This user-specific memory allows agents to provide more tailored and personalized responses, enhancing the user experience. User-specific memory is also reflected in that users can build knowledge bases for AI agents. This external memory source contains pre-defined information, facts, and answers. Just like the prior knowledge that everyone is born with, agents' knowledge bases help provide accurate and relevant responses to user inquiries.
Reasoning and Planning
When it comes to advanced cognitive abilities, agents and chatbots exhibit distinct patterns. Extensive research conducted on this subject reveals that the majority of chatbots primarily rely on "intuitive" System 1 responses, rather than engaging in more reflective cognitive processing (System 2). While the deployment of advanced large language models like GPT-4 and improvements in prompt engineering may enhance this aspect, the reasoning and planning capabilities of chatbots still fall considerably short of human levels. Conversely, AI agents demonstrate notable proficiency in reasoning and planning skills, equipping them to tackle complex tasks with efficiency. One key aspect is the ability to break down large tasks into smaller ones. This allows AI agents to tackle complex problems in a more organized and systematic manner. By breaking down the whole task, agents can focus on solving each subgoal individually, facilitating more manageable progress. This also allows the agent to allocate its resources effectively and prioritize the subgoals based on their importance or dependencies. Meanwhile, cognitive architectures empower AI agents to showcase reasoning abilities similar to human cognition. They serve as a blueprint for how AI agents process information, make decisions and perform tasks. Methods such as ReACT, CoT, and so on, contribute to enhancing the performance of AI agents on various tasks, often yielding impressive results.

Learning and Adaptation
It is also worth mentioning that AI agents have the ability to actively and continuously learn. AI Agents can learn from their experiences and adapt their behavior based on changing conditions. They can improve their performance over time through machine learning techniques. AI chatbots can also learn from user interactions. However, their focus is primarily on improving their language understanding and response generation, while they don't have the initiative to learn.
AI agents can utilize techniques such as online learning, incremental learning, and lifelong learning to adapt to changing environments and new information. Once the goal is set, they can actively seek out new data and update their models or algorithms based on the latest information. They can analyze real-time data, identify emerging patterns, and incorporate this knowledge into their decision-making processes.
An AI agent that possesses continual learning capabilities resembles a developing human expert. Driven by specific goals and motivations, it can progressively enhance its expertise and authority, enabling it to undertake increasingly complex and challenging professional tasks over time.
Metacognition
In this session, I would like to highlight the concept of metacognition. In humans, this high-order cognitive process is involved in almost all cognitive activities. Essentially, it involves our ability to be aware of and reflect upon our own thoughts and actions while engaged in various tasks. This metacognitive ability is largely absent in chatbots but can be observed in AI agents.
Frameworks like Reflexion enable AI agents to analyze their own performance, monitor their decision-making processes, and make adjustments based on self-reflection within a standard RL setup. Going into more detail, AI agents can evaluate the quality of their own decisions and assess the reliability of their models or algorithms. They can identify areas of uncertainty or limitations in their knowledge and take proactive measures to enhance their performance. They can analyze their own learning patterns, identify knowledge gaps, and adapt their learning strategies accordingly. Metacognition is an important part of human intelligence and human consciousness, and it serves as a crucial attribute that grants autonomy to AI agents. The inclusion of metacognition in AI systems opens up a world of possibilities. It enables AI agents to become more self-aware, adaptable, and accountable for their actions. This, in turn, can lead to advancements in various fields, such as healthcare, finance, and education.

AI agents vs AI chatbots: Applications
Those functional differences between AI agents and AI chatbots lead to different application scenarios. AI chatbots have found widespread applications in various fields, such as being deployed in search engines to enhance information retrieval and aggregation. They can understand the intent behind user queries, provide accurate search results, and continuously optimize the search experience based on user feedback. Besides, owing to their conversational capabilities, chatbots can serve as companions for chat and emotional support. Although they cannot replace professional psychologists or counselors, they can provide some comfort and guidance to users in need.
Although still in the nascent stage of development, AI agents hold tremendous potential across various industries. Their applications span fields such as autonomous vehicles, robotics, finance, healthcare, and cybersecurity. For example, autonomous vehicles use AI Agents to perceive their surroundings, analyze traffic patterns, and make decisions about navigation and safety. In the healthcare industry, AI agents can assist in diagnosing diseases, monitoring patient conditions, and recommending treatment plans.
What's more, AI agents have the potential to be deeply connected to our personal and professional lives. Experience the possibilities yourself by exploring MindOS, where a multitude of agents are readily available to assist. Users can witness the firsthand power of these agents and even create their own, tailoring them to their specific needs and preferences.
Conclusion
In this blog, we show that AI agents can be seen as an evolved version of AI chatbots, offering enhanced capabilities and functionalities. They possess a heightened level of autonomy in their actions and decision-making processes. They are able to operate independently, assess situations, and make informed decisions. This autonomy allows AI agents to actively engage with the environment and carry out tasks with reduced reliance on continuous human intervention.
While AI agents outperform AI chatbots in many ways, there is value in incorporating user-friendly interaction and emotional connections provided by chatbots into the deployment of agents. Better organic integration of agents with chatbots can not only advance AI technology but also facilitate user adaptation to the future AI ecosystem, where AI agents are set to become integral components of society.
As AI continues to advance, be it in the form of AI agents or AI chatbots, the pervasive adoption of AI technology will bring common ethical considerations, including privacy and data protection, bias and fairness concerns, alignment with human values, as well as transparency and accountability. As we navigate the path towards embracing AI on a larger scale, it is crucial to prioritize responsible and beneficial utilization of these technologies. By doing so, we can unlock the full potential of AI while ensuring the protection of individual rights and fostering a society that is fair and inclusive.
Related Readings:
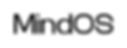