AI Research Highlights | Week 47, 2023
Updated: Feb 19

1. The Impact of Large Language Models on Scientific Discovery: a Preliminary Study using GPT-4
Source: https://arxiv.org/abs/2311.07361
In this report, Microsoft researchers delved into the performance of LLMs within the context of scientific discovery, focusing on GPT-4, the state-of-the-art language model. The investigation spans a diverse range of scientific areas encompassing drug discovery, biology, computational chemistry (density functional theory (DFT) and molecular dynamics (MD)), materials design, and partial differential equations (PDE). Preliminary exploration indicated that GPT-4 exhibits promising potential for a variety of scientific applications, demonstrating its aptitude for handling complex problem-solving and knowledge integration tasks.

2. Heuristics-Driven Link-of-Analogy Prompting: Enhancing Large Language Models for Document-Level Event Argument Extraction
Source: https://arxiv.org/abs/2311.06555
In this work, authors hypothesized and validated that LLMs learn task-specific heuristics from demonstrations via in-context learning, which can provide a guidance on the example selection process. Building upon this hypothesis, they introduced an explicit heuristic-driven demonstration construction strategy, and proposed a link-of-analogy prompting method. Extensive experimentation reveals that the HD-LoA prompting not only outperforms cuttingedge prompting methods and few-shot supervised methods in document-level EAE task, but also exhibits effectiveness across various non-reasoning NLP tasks.

3. It's Not Easy Being Wrong: Evaluating Process of Elimination Reasoning in Large Language Models
Source: https://arxiv.org/abs/2311.07532
The researchers from University of Maryland proposed PoE with COT, a new task where LLMs must reason toward incorrect options on multiple-choice questions. And the results showed that PoE consistently underperforms directly choosing the correct answer. The agreement of these strategies is also lower than the self-consistency of each strategy.

4. On the Discussion of Large Language Models: Symmetry of Agents and Interplay with Prompts
Source: https://arxiv.org/abs/2311.07076
In this study, researchers explored various dimensions of combining prompt engineering and discussion mechanisms to improve reasoning abilities of language models. (1) They proposed a theoretical framework to systematically characterize discussion engineering based on the concept of discussion mechanisms symmetry. (2) Experiments showed that reasoning capability of language models can be enhanced by either a strong prompt but simple mechanism or a strong mechanism but a simple prompt. (3) They also proposed a conquer and merge style (CMD) mechanism of group discussion, which is more scalable and outperforms other discussion mechanisms in many settings.

5. From Complex to Simple: Unraveling the Cognitive Tree for Reasoning with Small Language Models
Source: https://arxiv.org/abs/2311.06754
In this paper, researchers proposed the Cognitive Tree (CogTree) framework, consisting of the Intuitive System and the Reflective System. The Intuitive System and the Reflective System are both generative models, albeit with distinct objectives. The Intuitive System employs in-context examples to dissect intricate problems into sub-problems and produce responses to the query. Conversely, the Reflective System evaluates the outcomes generated by the Intuitive System and chooses the most likely solution to provide guidance for the next generation step. The aforementioned process is an iterative tree generation process, which continues until the original problem is decomposed into manageable sub-problems (corresponding to nodes on the tree) that can be easily solved.

6. Think-in-Memory: Recalling and Post-thinking Enable LLMs with Long-Term Memory
Source: https://arxiv.org/abs/2311.08719
In this paper, researchers from Ant Group proposed a novel human-like long-term memory mechanism called Think-in-Memory (TiM), enabling LLMs to remember and selectively recall thoughts. TiM can let LLM think in memory without repeated reasoning over the long-term history. And they formulated some basic principles to organize thoughts in memory based on well-established operations, which mirrors human cognitive processes to empower dynamic updates and evolution for thoughts in memory. Besides, a hash-based retrieval mechanism is introduced for efficient utilization of TiM. The experiment results indicated that our method can substantially enhance LLM’s performance across various dimensions: (1) It enables diverse topics ranging from open to specific domains; (2) It supports bilingual languages in both Chinese and English; (3) It improves response correctness and coherence.

7. Chain-of-Note: Enhancing Robustness in Retrieval-Augmented Language Models
Source: https://arxiv.org/abs/2311.09210
Researchers from Tencent AI lab introduced Chain-of-Noting (CoN), a novel approach aimed at improving the robustness of RALMs in facing noisy, irrelevant documents and in handling unknown scenarios. The core idea of CoN is to generate sequential reading notes for retrieved documents, enabling a thorough evaluation of their relevance to the given question and integrating this information to formulate the final answer. Experiments across four open-domain QA benchmarks show that RALMs equipped with CoN significantly outperform standard RALMs.

8. Contrastive Chain-of-Thought Prompting
Source: https://arxiv.org/abs/2311.09277
In this paper researchers analysed various invalid reasoning types and find that combining positive and negative demonstrations generally boost the effectiveness of chain-of-thought. Thus, they proposed contrastive chain of thought to enhance LLM reasoning. To improve generalization, they also proposed an automatic method to construct contrastive demonstrations. Evaluations on multiple reasoning benchmarks demonstrate significant improvements compared to conventional chain of thought. The code will be released here.

9. Think Before You Speak: Cultivating Communication Skills of Large Language Models via Inner Monologue
Source: https://arxiv.org/abs/2311.07445
Researchers from CAS endowed LLMs with communication skills and inner monologue (CSIM) through prompt engineering and in-context learning, making LLMs more anthropomorphic and proactive. They also proposed a benchmark Cskills for evaluating various communication skills. Evaluations showed that CSIM improved backbone models and outperformed baselines.

10. Think Twice: Perspective-Taking Improves Large Language Models' Theory-of-Mind Capabilities
Source: https://arxiv.org/abs/2311.10227
The researchers from Carnegie Mellon University proposed a two-prompt approach to eliciting Theory-of-Mind capabilities in LLMs by first asking LLMs to perspective-take: to filter context to what the character in question knows. The results showed that LLMs are substantially stronger at ToM reasoning than they appear when probed with a single inference pass.

*The researchers behind the publications deserve full credit for their work.
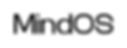